News Item
Above: Barbara Biasi (Yale SOM) presented ' What Works and For Whom? Effectiveness and Efficiency of School Capital Investments Across The U.S. ' at the Labor & Public Economics Conference. Each summer the Cowles Foundation brings together leading …
Relevance: 4.4175673
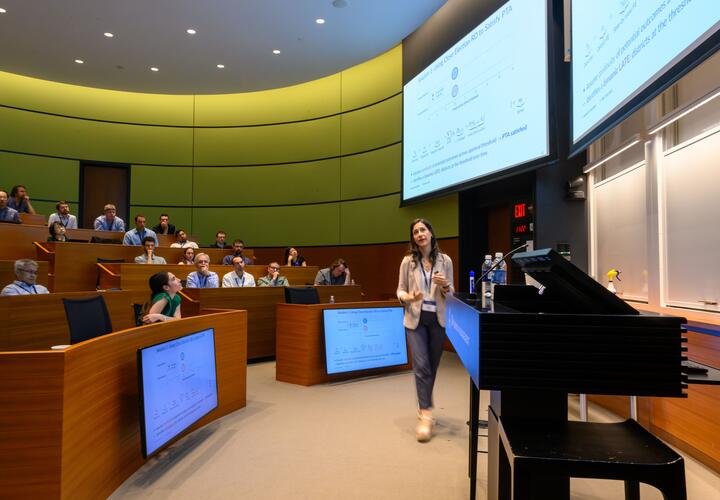