News Item
Amanda Kowalski has been awarded the Arthur Greer Memorial Prize for her outstanding scholarship in social sciences. In an email sent to Kowalski from Alan Gerber, one of the committee members, he said “The committee was extremely impressed with your …
Relevance: 2.7621388
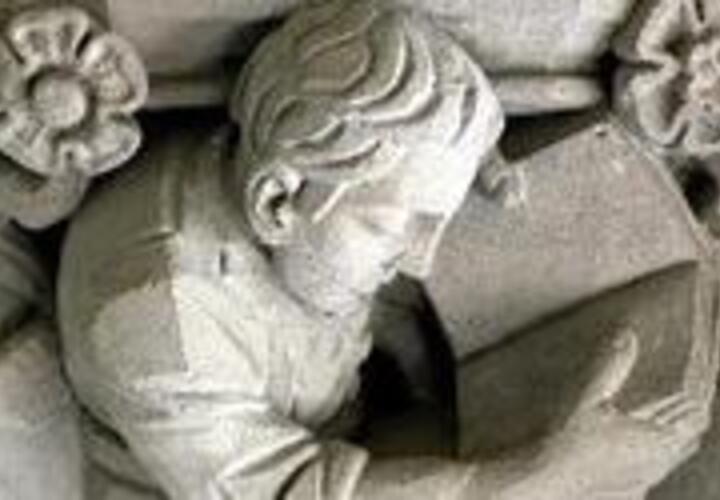